Read next
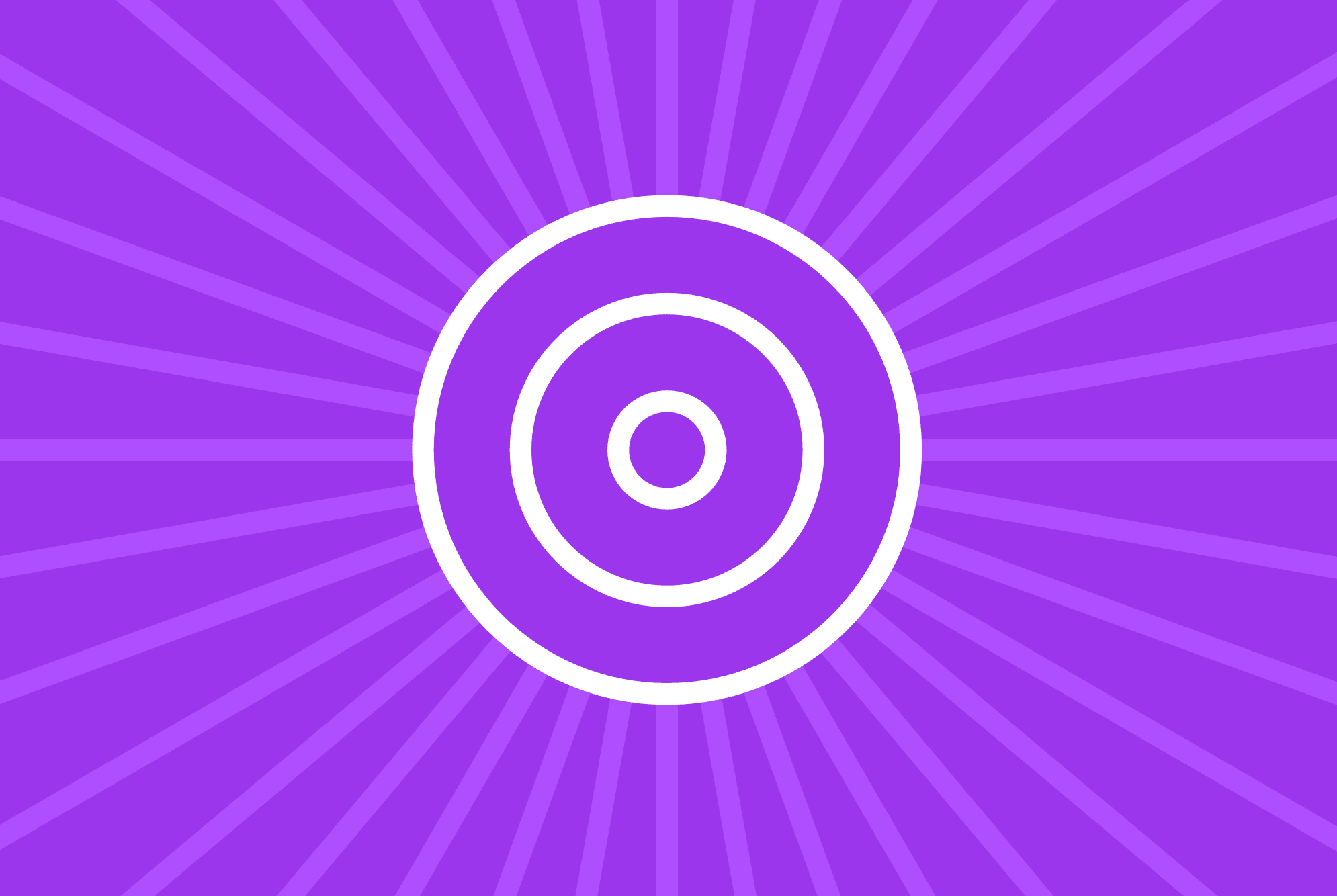
Targeting Customers Who Are Likely to Buy
Improving Targeting & Predicting Response to Marketing Campaigns
Read ArticleThe Challenge:
Working with a top 25 Insurance Carrier, we were challenged to improve customer retention efforts by accurately identifying policy holders who are most likely to move their policy to a competitor at renewal.
The Solution:
The Insurance Carrier analyzed over 200 Powerlytics variables to determine which were most predictive of customer retention. Using these new variables, they created a new customer retention model and compared it with their existing model to see which delivered the greatest ability to predict who was most likely to leave and who was most likely to stay. This information would then allow them to focus their customer retention efforts on those areas delivering the biggest return on investment.
By scoring a blind sample, the current model is able separate the policies into customer groups that are most loyal (92.4%) to customer groups that are most likely to leave the insurance carrier (55.6%). The lift derived from the current model is 36.8 points.
By scoring the same blind sample of policies through the model using Powerlytics variables, they were able to identify the most loyal customer groups (94.0%) and those most likely to leave the insurance carrier (54.3%) This model provides a greater lift of 39.7 points.
The Powerlytics model is able to identify a greater number of “at risk” policies, while also being able to better identify which ones were not as likely to attrite. With identical proactive and assertive retention strategies, they estimated that the model would result in a 4.3% incremental policy growth above the current model.
The Impact:
While both models predict well, the model adding the Powerlytics variables provided greater lift, less predictive error and more incremental value than the existing model.
Using the data collected, they projected the incremental value of using the Powerlytics data to their book of business with 2 million policies would be approximately 3,800 policies. With an average annual premium of $1,000 this would result in saving approximately $3.8 million in premiums.
Improving Targeting & Predicting Response to Marketing Campaigns
Read Article